Neil Gaikwad
नील गायकवाड
Assistant Professor
HCI Researcher focused on Human-AI Value Alignment, Policy, and Sustainability
School of Data Science and Society, Department of Computer Science
University of North Carolina, Chapel Hill
Faculty Advisory Council, UNC Parr Center for Ethics, Department of Philosophy
Fellow, MIT Dalai Lama Center for Ethics and Transformative Values
Ph.D. Massachusetts Institute of Technology
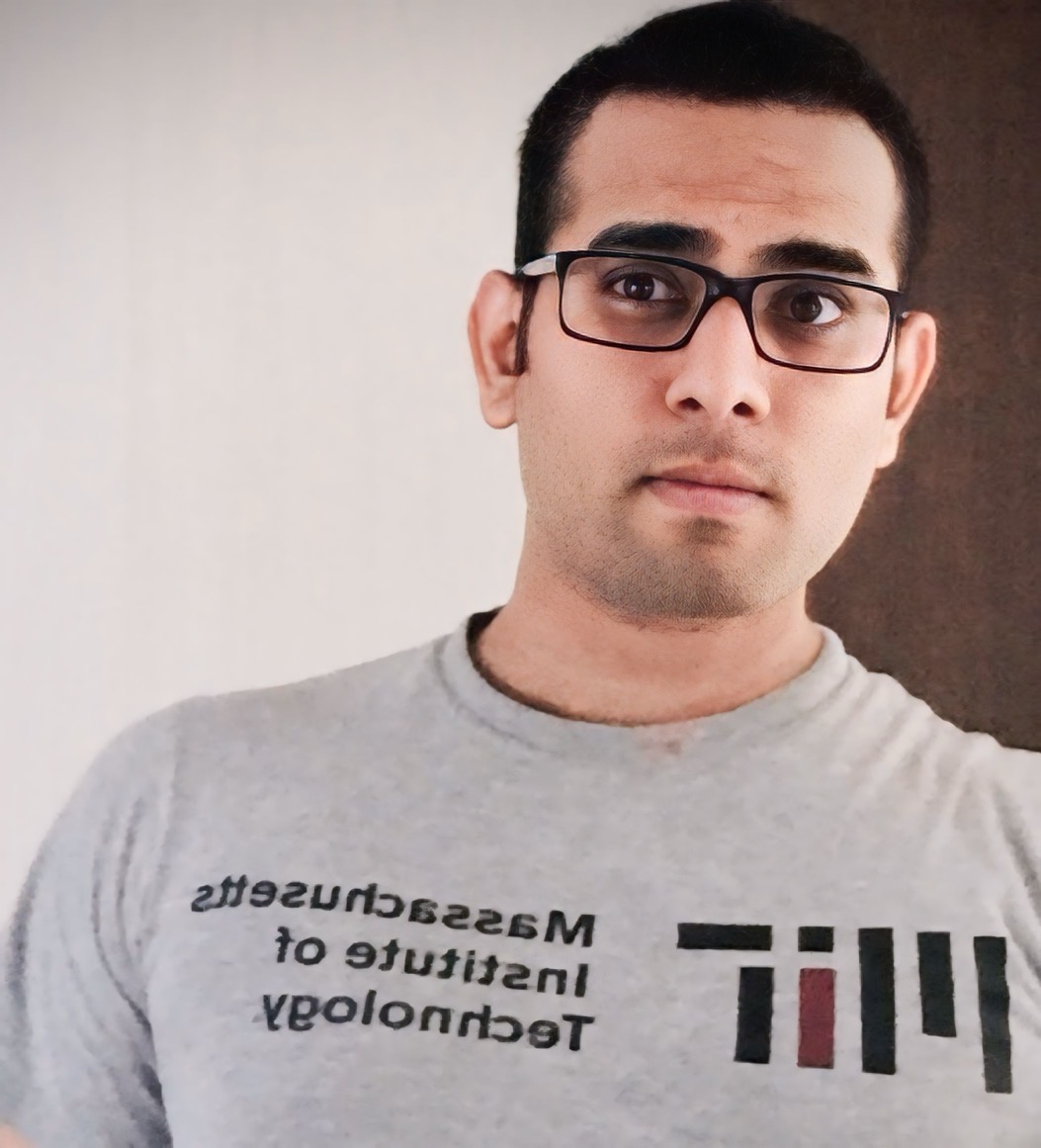
I have received my PhD in Society-Centered AI from MIT where I was advised by Danielle Wood, Julie Shah, Sally Haslanger, Bish Sanyal, and Nabeel Gilani. I joined the University of North Carolina, Chapel Hill as an Assistant Professor in Data Science and Computer Science, starting in Fall 2024. If you are interested in joining my lab as an undergrad, PhD student, or postdoc, feel free to drop me an email. For PhD positions, consider applying to the UNC Computer Science, Information Science, or Statistics & Operations Research programs, and be sure to mention my name in your application.
The rise of AI has fundamentally altered the relationship between algorithms and ethical values, transforming both computing and society. As a researcher in human-computer interaction and ethical machine learning, I focus on Human-AI Alignment and Policy to drive equitable societal development, particularly for underserved global populations. My work creates novel, value-sensitive designs for interactive systems that integrate ethics with engineering principles, aiming to promote fair decision-making at individual and institutional levels. This public interest research examines the impact of algorithmic systems on societal disparities and development trajectories within broader sociotechnical contexts. Through equity-focused analysis of AI-driven processes, I develop systems to enhance collective decision-making and broaden access to socioeconomic opportunities for diverse populations. The work extends to policy domains, addressing issues such as algorithmic fairness, poverty reduction, climate change mitigation, and public health. Ultimately, this research aims to create inclusive sociotechnical approaches that benefit marginalized communities globally.
I am a fellow at MIT's Dalai Lama Center for Ethics and Transformative Values. By establishing grassroots community partnerships and contributing to policy discussions, I strive to address societal challenges comprehensively and improve equitable AI governance at various levels. My commitment to broadening equitable access to STEM and policy education is reflected in research, science policy initiatives, mentoring, and teaching at UNC, CMU, and MIT (EECS, IDSS, and Media Lab). I've mentored over 30 students from diverse backgrounds, supporting their publication of influential papers on equity in AI, securing fellowships, pursuing research careers, and shaping AI fairness policies. These efforts were recognized with MIT's Karl Taylor Compton Prize.
Recent Talks & Travels NeuirIPS 2024
Stanford University
National Science Foundation
University of Michigan, Ann Arbor
UW Seattle, CSE CHANGE Seminar
Carnegie Mellon
UNC Chapel Hill
Cornell University
Imperial College London
Teaching Data 120 (UNC): Ethics of Data Science and AI (200 students)
6.3952 (MIT EEES): AI, Decision-Making, and Society (212 students)
6.UAR (MIT EEES): Undergraduate Advanced Research (81 students)
IDS 412J (MIT IDSS): Science, Technology, and Public Policy (80 students)
Program Committee/Session Chair ACM/AAAI Conferences 2023/2024 ACM FAccT
EAAMO (Equity & Access in Algorithms, Mechanisms, & Optimization)
AIES (AI, Ethics, and Society)
Research
Neil Gaikwad holds PhD from the Massachusetts Institute of Technology, specializing in Society-Centered AI. Neil received a master's degree from the School of Computer Science at Carnegie Mellon University, where he worked at the Robotics Institute and Software and Societal Systems Department. He was also a part of the Complex Systems Summer School at the Santa Fe Institute. Before MIT, Neil worked extensively on machine learning and decision-making research in his job as a data scientist in financial industry.
Neil leads the development of AI and Policy research program (Gaikwad et al., 2020, 2021, 2022). His research has been published in artificial intelligence and human-computer interaction conferences (AAAI, ACM CSCW, ACM UIST, ACM CHI) and a scientific journal (PNAS), and also featured in the New York Times, Bloomberg, WIRED, and The Wall Street Journal. Neil, along with his colleagues, has built the Ethical AI system that learns a model of high-stake societal decision-making from 1.3 million people. He is also one of the principal creators and founding members of Daemo, a self-governed AI data market, and EteRNA, a crowd computing system that enables massive scale human and machine collaboration to realize scientific discoveries neither of them can accomplish in isolation. He led the open source development of Daemo's sociotechnical architecture that demonstrates mechanisms for designing equitable AI data market systems (Gaikwad et al., 2015, 2016, 2017). His foundational contributions draw on mechanism design (incentive-compatible reputation systems for AI data markets), portfolio management (tranches-based guilds for the future of work), and human-centered design (shared mental model of task composition in AI data markets).
Neil's research has resulted in deployable open-source systems, environmental art exhibitions, and technical talks at global policymaking forums of the United Nations and European Union. His scholarship continues to benefit researchers and practitioners worldwide. Daemo has been cited in the International Labour Organization's (ILO) report on Digital Labour Platforms and the Future of Work and deployed to create SQuAD, Stanford's Natural Language Processing dataset. Besides, Boomerang (Gaikwad et al. 2016) has been a part of the advanced HCI/human-computation course curriculum at leading universities. EteRNA has reached over 250,000 citizen scientists as of 2016; it was also one of the 25 great ideas from CMU's School of Computer Science. Neil's TEDx talk presents his vision for just market institutions in the era of AI.
Neil is committed to increasing diversity and belonging in science, technology, and policy. He was one of the core members of the Stanford Crowd Research Initiative, MIT's Innovating for Billions in Emerging Worlds Leadership Council, and Computational Sustainability Open Graduate Seminar organizers. He has mentored over 20 students, who have gone on to win prestigious awards (e.g., Apple Ph.D. Fellowship), pursue careers in research, and publish influential scholarship that has shifted the discourse on AI fairness. Neil's research, teaching, and professional service have been widely recognized with the Facebook Research Ph.D. Fellowship, William Asbjornsen Albert Memorial Fellowship (awarded to one MIT student conducting research in modern science and engineering), UChicago Rising Stars in Data Science, MIT GSC Graduate Teaching Award (presented annually to one MIT professor or teaching assistant from each school for excellence in teaching a graduate-level course).
Selected Awards and Honors
Karl Taylor Compton Prize, 2019, MIT's Highest Student Award
Research and Innovation
Facebook Research Fellowship, 2019
Rising Star in Stanford Management Science and Engineering, 2024
PKG Fellowship for Public Interest Tech and Social Impact, 2024
Schmidt Science Fellowship MIT Nomination, 2023
Fellow, MIT Center for Ethics and Transformative Values, 2023
MIT Social & Ethical Responsibilities of Computing Scholar, 2023
Rising Stars in Data Science, UChicago, 2021
MIT William Asbjornsen Albert Memorial Science & Engg Fellowship, 2021
MIT Human Rights & Technology Fellowship, 2021
INK Fellow Emerging Innovator, 2019
Best Paper Honorable Mention, UIST 2017
Teaching
MIT GSC Graduate Teaching Award, 2018
MIT Kaufman Teaching Certificate, 2017
Teaching Fellow MIT EECS Comm Lab, 2020
Professional Service
EAAMO 2021, Program Committee, Best Reviewers
CHI 2020 and CHI 2019 Special Recognition, Outstanding Reviews
IJCAI 2018, Distinguished PC
Arts
MIT Arts Scholars
National Geographic Photo of the Day
Publications (Organized by Research Themes)

Annual Ethical AI Symposium 2024, The Michigan Institute for Data Science, University of Michigan, Ann Arbor
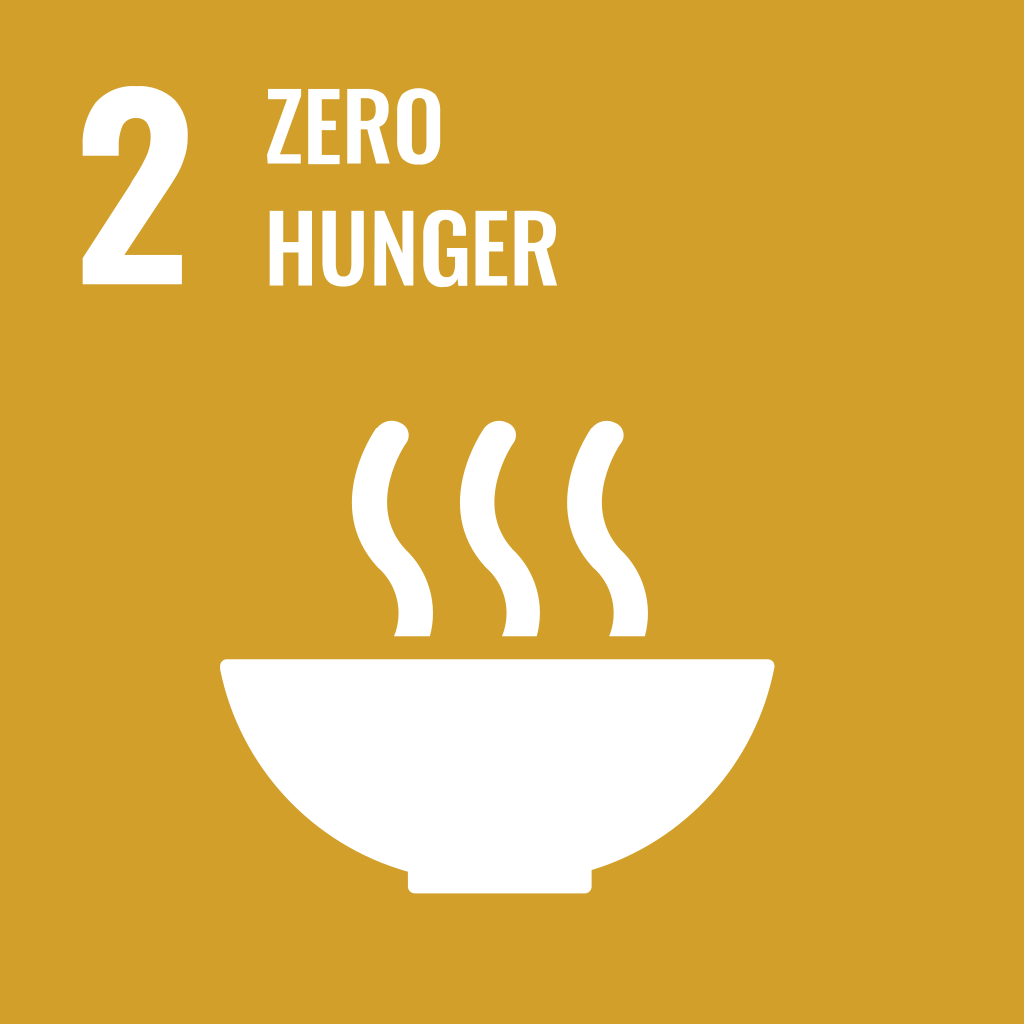
The National Science Foundation (NSF) Workshop on Sustainable Computing 2024
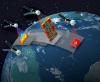
KDD 2022, KDD 2021, KDD 2020: ACM SIGKDD Conference on Knowledge Discovery and Data Mining. link website
picture_as_pdf paper 2022
picture_as_pdf paper 2021
picture_as_pdf KDD workshop proceedings 2021
picture_as_pdf KDD workshop proceedings 2020
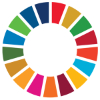
Santa Fe Institute, Complex Systems Summer School Proceedings 2018
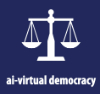
AAAI 2018: Association for the Advancement of Artificial Intelligence (Acceptance rate of 24.6%)
picture_as_pdf aaai paper book journal version in R&R state bookmarkgoogle scholar Press: "Social Media Has Failed Its Self-Driving Test"(Bloomberg) open_in_new"Researchers Go After the Biggest Problem with Self-driving Cars"(Axios) open_in_new
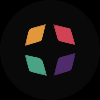
❊Correspondence authors, *equal Contributions.
CSCW 2019: The ACM Conference on Computer-Supported Cooperative Work and Social Computing
picture_as_pdf paper bookmarkgoogle scholar
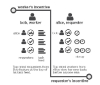
UIST 2016: The ACM Symposium on User Interface Software and Technology, (Acceptance rate of 20.6%)
picture_as_pdf paper code open source software system bookmarkgoogle scholar bookmark In the course curriculum of Human Computation and Human-computer Interaction classes at Cornell University (CS 5306/INFO 5306), KAIST (CS492), UW Seattle (CSE590H)
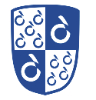
CSCW 2017: The ACM Conference on Computer-Supported Cooperative Work and Social Computing (Acceptance rate of 25%) picture_as_pdf paper bookmarkgoogle scholar

CSCW 2017: The ACM Conference on Computer-Supported Cooperative Work
picture_as_pdf paper code open source software system bookmarkgoogle scholar

UIST 2015: The ACM Symposium on User Interface Software and Technology
picture_as_pdf paper picture_as_pdf poster code open source software system bookmarkgoogle scholar Press: "The future of work the peoples uber"(Pacific Standard) open_in_new
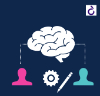
AAAI HCOMP 2017: AAAI Conference on Human Computation
picture_as_pdf paper code open source software system bookmarkgoogle scholar
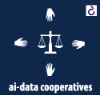
Collective Intelligence 2017: ACM Collective Intelligence Conference
picture_as_pdf paper code open source software system bookmarkgoogle scholar
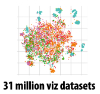
CHI 2019: The ACM CHI Conference on Human Factors in Computing Systems (Acceptance rate of 23.8%) picture_as_pdf paper code open source software system bookmarkgoogle scholar
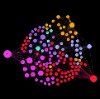
IC2S2 2017: International Conference on Computational Social Science
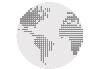
Belongie, Serge., Goel, Sharad., Davis, James., and Bernstein, Michael.
UIST 2017: ACM Symposium on User Interface Software and Technology, (Acceptance rate of 22.5%)
picture_as_pdf paper link website bookmarkgoogle scholar
emoji_events ACM UIST Best Paper Honorable Mention, top 2.5% of the technical paper submissions
Design Thinking Research: Looking Further: Design Thinking Beyond Solution-Fixation
Springer Nature 2019
book book chapter bookmarkgoogle scholar Press: "A Stanford-led Platform for Crowdsourced Research Gives Experience to Global Participants"(Stanford News) open_in_new
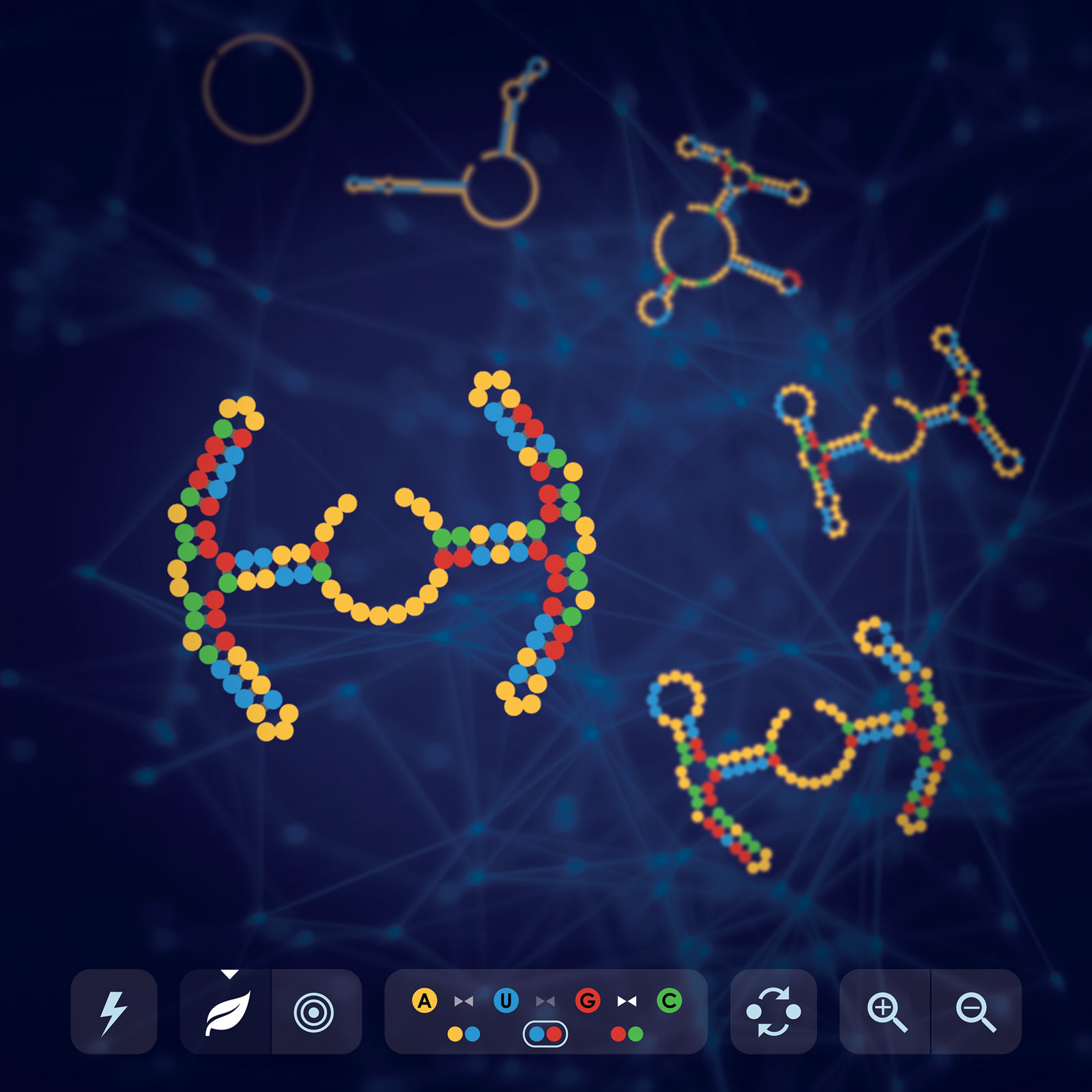
Gaikwad, S., Yoon, Sungroh., Treuille, Adrien., Das, Rhiju., and EteRNA Participants
PNAS 2014: Proceedings of the National Academy of Sciences of the United States of America
picture_as_pdf paper code software system bookmarkgoogle scholar "Videogamers are recruited to fight Tuberculosis and other ills "(Wall Street Journal) open_in_new "RNA game lets players help find a biological prize"(New York Times) open_in_new "How turning science into a game rouses more public interest"(WIRED) open_in_new "Game lets citizen scientists participate in creating large-scale library of synthetic RNA designs"(Scientific American) open_in_new "Why video games are key to modern science "(CNN) open_in_new "Online game helps predict how RNA folds " (New Scientist) open_in_new
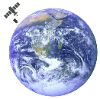
CSCW 2020: The ACM Conference on Computer-Supported Cooperative Work and Social Computing
picture_as_pdf paper link website bookmark Exhibited in the Ars Electronica 2020 Festival of Arts, Technology, and Society, as a part of the Garden CAMBRIDGE
AI Tech Policy Engagements and Impact
Founded an AI and Policy initiative to address technological and sustainability challenges with data-driven policymaking, bridging the research-to-policy gap.
Designing Human-centered AI for Socioeconomic Development
Invited Talk and Panel: United Nations AI for Good Summit, Geneva, Switzerland, 2019
How artificial intelligence is transforming the world
Brookings Institution: Citation of A Voting Based System for Ethical Decision Making by Darrell West and John R. Allen in AI Policy Report, 2018
The Brookings Institution is a nonprofit organization whose mission is to conduct in-depth, nonpartisan research to improve policy and governance at local, national, and global levels.
ILO Report leverages Daemo (Gaikwad et al., 2016) and Boomerang (Gaikwad et al., 2017), utilizing scientific findings as evidence to shape and validate policy recommendations aimed at enhancing working conditions within the digital labor platform ecosystem.
International Labor Organization’s (ILO) Digital Labour Platforms and the Future of Work Report, 2018
The International Labour Organization is a United Nations agency whose mandate is to advance social and economic justice by setting international labour standards.
AI for Social Good National Initiative
Invited as part of a group of select computer scientist to the inauguration ceremony of Wadhwani AI Institute launched by
the Prime Minister of India, Chief Minister of Maharashtra State, and the CEO of NITI Aayog Policy Think Tank, 2018
Daemo, a Self-governed Crowdsourcing Market with an Incentive Compatible Reputation System
Invited Talk: A European Dialog on the Platform Economy, European Trade Union Institute and Partners, Brussels, Belgium, 2018
Human-centered AI and Policy for Social Good, MIT Emerging Worlds Initiative
Federation of Indian Chambers of Commerce & Industry (FICCI), Delhi, India, 2017
NITI Aayog Policy Commission of India and FICCI Delegation of Women Entrepreneurs, Boston, 2018
MIT Policy Hackathon 2023 (260 participants from all around the world)
Tech Policy Hackathon, Technology and Policy Program at MIT's Institute for Data, Systems, and Society
Please refer to the teaching section for courses on AI and Policy
Teaching
Course Topics: Classroom Teaching and Course Evaluations at MIT EECS, MIT IDSS, MIT Media Lab
6.3952 AI, Decision-Making, and Society, MIT EECS (Electrical Engineering and Computer Science), Fall 2023
subject Number of Students: 212 | Student Evaluations: 7/7
subjectGraduate Teaching Assistant and Recitation Instructor for a course in MIT's major 6-4. Taught a lecture on Ethical AI and Policy
subject Instructors: Ashia Wilson and Manish Raghavan
subject Student Evaluation Quotes:
(1) “[...] Neil is easily the single most influential course staff, and mentor, I’ve ever interacted with at MIT. [...]”
(2) “Best TA I’ve ever had! So kind, supportive, and helpful, both in and out of the classroom. [...]”
(3) “Amazing TA! Very thoughtful and cared about us students as people. Willing to meet and help outside of typical times and generally went above and beyond.”
(4) “[...] The slides were helpful as well as beautiful. It felt like Neil had a vested interest in how we were developing as students. He stimulated group discussions well and kept the conversations productive.”
(5) “Very kind and wonderful TA! Goes out of his way to help students learn. Did an excellent job!”
IDS.412 Science, Technology and Public Policy, MIT IDSS (Institute for Data, Systems, and Society), Spring 2023
subjectNumber of Students: 80 | Student Evaluations: 6.5/7
subjectGraduate Teaching Assistant and Recitation Instructor for a Core course on methods and frameworks for evidence based policy-making. Taught a lecture on AI and Policy
subject Student Evaluation Quotes:
(1) “Neil was a star. He is the only reason I was able to comprehend any of the information in this class. [...] He taught us the questions we should be asking and how to approach problems. He genuinely cared for our wellbeing and I am grateful to him for all his hard work.”
(2) “Neil went above and beyond both in teaching core concepts and making sure we feel supported both in and out of the classroom. [...]”
(3) “[...] I can’t imagine getting through the class without his recitation sessions. [...]”
6.UAR: Undergraduate Advanced Research, Spring 2024
subjectNumber of Students: 81 | Student Evaluations: 6.8/7
subjectGraduate Teaching Assistant and Project Mentor
subject Instructor: Dina Katabi
MAS.S61 Computational/Quantitative Social Science Research Methods, MIT Media Lab, Fall 2017
subjectStudent Evaluations: 7/7
subjectGraduate Teaching Assistant, Data Science Lab Instructor, & Course Co-Designer
emoji_events MIT GSC Graduate Teaching Award (presented annually to one MIT professor or teaching assistant from each school for excellence in teaching a graduate-level course)
IC2S2 Conference Tutorial Crowdcomputing and Citizen Science for Large-Scale Experiments, 2017
subjectLead Instructor and Course Designer
Carnegie Mellon 15 − 859 (B) Machine Learning Theory, Computer Science Department, Spring 2010
subjectCourse Grader
MIT Emerging Worlds: Introductory Seminar Course on Machine Learning for Development, 2017-2018
subjectInstructor, Innovation Mentor, and Seminar Designer
MIT Emerging Worlds: Design Thinking and Innovation Workshop for Women Entrepreneurs, 2018
subject Lead instructor for the workshop
MIT 15.376/MAS.664: AI for Impact: Towards Solving Societal-Scale Problems, MIT Media Lab+MIT Sloan School of Management Course, Spring 2020
subject Course Project Mentor
Carnegie Mellon 45 − 881: Entrepreneurial Thought & Action, Tepper School of Business, Spring 2009
subject Teaching Assistant
MIT 15.226 Modern Business in India: MIT Sloan School of Management Course, 2019
subjectGuest Lecture: Human-centered Machine Learning for Socioeconomic Development
MIT Emerging Worlds: Design Thinking and Innovation Workshop for Executive Program in General Management Course at MIT Sloan School of Management, 2017
subject Lead instructor for the workshop
MIT EECS 6.S899 Special Subject Computer Science, 2020
subject Teaching Fellow, MIT EECS Communication Lab
subjectStudent Evaluations: 7/7
Navjyoti India Foundation, India Remedial Education Program, 2018
subject Guest Lecture for class 10th remedial education students
100 Robots for 100 Kids, 2010
subject Robotics Teacher Volunteer for a grass roots project to inspire Pittsburgh's at-risk children about computing and robotics
Andrew’s Leap, a Summer Enrichment Program for High School Students, the Carnegie Mellon School of Computer Science, 2010
subject Co-instructor for the Game Play session of EteRNA
Selected Talks, Demos, & Panel
Topics: Socially and Ethically Responsible AI, Public Policy, Sustainability and International Development
Public Interest Computing: Pluralistic Design of Ethically Responsible AI and Policy
University of Washington, Seattle, CHANGE Seminar, 2023
Public Interest Computing: Pluralistic Design of Ethically Responsible AI and Policy
Carnegie Mellon University, 2023
UNC Chapel Hill, 2023
Cornell University , 2023
Imperial College London, 2023
Pluralistic Design of Ethical AI and Policy for Sustainable Development
International College of Innovation, National Chengchi University, Taiwan, Taipei City, 2023
Community-based Designs of Human-centered AI and Policy: Towards Inclusion, Resilience, and Sustainability
Schmidt Science Fellows Global Meeting, 2022
University of Chicago, Data Science Institute, 2021
Data-driven Humanitarian Mapping Research and Policy Initiative
KDD Data-driven Humanitarian Mapping Workshop, 2021
Increasing Equity in Agricultural Markets Under Climate Shocks through AI and Participatory Policy
Harvard University, Weatherhead Center for International Affairs, Global FOOD+2021
Human-centered AI for Socioeconomic Development
AI for Social Good, Center for Research on Computation and Society, Harvard University, 2020
Designing Human-centered AI for Socioeconomic Development
United Nations AI for Good Summit, Geneva, Switzerland, 2019
Human-centered Machine Learning for Socioeconomic Development
Oak Ridge National Laboratory, Tennessee, 2019
Challenges and Opportunities in Designing Human-centered Artificial Intelligence Systems
Dell EMC, Massachusetts, 2019
Daemo, a Self-governed Crowdsourcing Market with an Incentive Compatible Reputation System
A European Dialog on the Platform Economy, European Trade Union Institute and Partners, Brussels, Belgium, 2018
Human-AI Collaboration for Sustainable Market Design: A Capability-Based Realization Focus Perspective
Harvard Berkman Klein Center and Media Lab, Ethics and Governance of AI, 2018
Design Thinking and Crowd Research: Open and Scalable University Laboratories
The HPI School of Design Thinking, Hasso-Plattner-Institut Germany, HPI-Stanford Design Thinking Summit, 2017
The Science of Human Computation
Xerox Research, Webster, NY 2014
The Future of Markets in the Era of Artificial Intelligence TEDx, BeaconStreet Boston
The Future of Food Security, 2019
PopTech and RISD, Providence
Artificial Intelligence, Ethics, and Social Good, 2018
Wadhwani Institute for Artificial Intelligence, Mumbai, India
Panel on Crowdsourcing,declined to increase the panel’s gender diversity
AAAI Human Computation and Crowdsourcing (HCOMP 2017), Québec City, Canada
AI Fairness, Societal Impact, and Governance, CRCS Rising Stars Workshop on AI for Social Impact, 2020
Panelists: Milind Tambe, Cynthia Dwork, Jonathan Zittrain
Human-centered AI for Music and Immersive Experience, 2018
Demo to A. R. Rahman, Grammy and Academy Award Winning Musician
Human-centered AI and Storytelling, 2018
Demo to Shekhar Kapur, an international film director, actor, and producer
Professional Service, Leadership, and Initiatives
Data Science, AI Ethics, and Computational Sustainability
subject ACM FAccT [2024]: ACM Conference on Fairness, Accountability, and Transparency
subject ACM EAAMO [2024, 2023, 2022, 2021 emoji_events]: ACM Conference on Equity and Access in Algorithms, Mechanisms, and Optimization
subject AAAI AIES [2024, 2023]: AAAI Conference on AI, Ethics, and Society
subject ACM COMPASS [2022]: ACM Conference on Computing and Sustainable Societies
subject IC2S2 [2021]: International Conference on Computational Social Science
subject SIGKDD DHM [2022, 2021, 2020]: Data-driven Humanitarian Mapping Workshop
subject AAAI [2020]: Conference on Artificial Intelligence
subject NeurIPS ML4PH [2021]: Machine Learning in Public Health Workshop
subject NeurIPS ML4D [2021, 2020, 2019]: Machine Learning for Development (ML4D) Workshop
subject IJCAI [2018 emoji_events]: International Joint Conference on Artificial Intelligence
Human-computer Interaction and Social Computing
subject ACM Creativity & Cognition [2024]: ACM Conference on Creativity and Cognition
subject ACM CHI [2023, 2020 emoji_events , 2019 emoji_events ]: ACM Conference on Human Factors in Computing Systems
subject ACM CSCW [2020 LBW, 2019, 2018]: ACM Conference on Computer-Supported Cooperative Work and Social Computing
subject WWW [2017]: International World Wide Web Conference
subject Invited Judge, MIT $100K Entrepreneurship Competition, Accelerate (2022)
subject MIT Media Lab, Diversity, Inclusion, and Equity Committee (2000-2021)
subject Co-chair, MIT SP Committee on Scholarly Interactions, (CoSI), a graduate student organization dedicated to stimulate and foster intellectual exchanges among the MIT community (2016-2020)
subject Co-Chair, MIT India Conference
subject Leadership Council, MIT Innovating for Billions Initiative
subject Media Lab Design Rep, GradRat Ring
subject Reviewer Committee, MIT Graduate Student Council Travel Grant
subject Co-organizer, EteRNA sessions for Leap@CMU Summer enrichment program for high school students
subject Lead & co-founder, Data-driven Humanitarian Mapping: Data to Global Policy Research Initiative
subject Lead and co-founder, MIT Graduate Student Symposium Series
subject Founder Undergraduate Research Mentoring Initiative, a bridge between the MIT UROP program and postdoc, graduate, and undergraduate communities
subject Lead Founder Science, Technology, and Social Justice Speaker Series, MIT SP Committee on Scholarly Interactions
Organizations
subject Program Committee and co-organizer Computational Sustainability Open Graduate Seminar, (with doctoral students from Cornell, Harvard, GeorgiaTech, CMU, USC, and UMass Amherst)
subject Program Committee & co-organizer The Harvard Center for Research on Computation and Society, Global Workshop on AI for Social Impact open_in_new
subject MIT Sidney Pacific House Government (2016-2020)
subject 100 Robots for 100 Kids, a grass roots project to inspire Pittsburgh's at-risk children (at Carnegie Mellon Robotics Institute)
Student Supervision, Guidance, & Mentoring
subject Jack Forman, MIT Media Lab (MIT William Asbjornsen Albert Memorial Fellowship)
subject Dishita Turakhia, MIT EECS (Facebook Ph.D. Fellowship)
subject Ziv Epstein, MIT Media Lab (Publication at the ACM CHI)
subject Joy Buolamwini, MIT Media Lab (Publication at the ACM FAccT)
subject Ishwarya Ananthabhotla, MIT Media Lab (Apple Scholars Ph.D. Fellowship in Machine Learning)
M.S. students at MIT
subject Eunseo Choi, MIT Computer Science and Technology Policy (Publication at the ICML Generative AI + Law Workshop)
subject Ankur Podder, Architecture Studies (SMArchS) - Urbanism
subject Xuenan Ni, MIT Department of Urban Studies and Planning
Undergraduate Students at MIT, Harvard, Wellesley College
subject Kateryna Morhun, MIT EECS (Selection in the AI Research Workshop, Johns Hopkins University Center for Language and Speech Processing)
subject Nikhil Behari, Harvard CS (Publication at the ACM KDD Data-driven Humanitarian Mapping Workshop)
subject Yodahe Alemu, MIT EECS
subject Kealani Finegan, MAS Wellesley College
subject Sourav Das, MIT EECS
subject Alice Jin, MIT EECS, Co-supervised
MIT 6.UAR Super UROP Students
subjectAdithya Balachandran, MIT Mathematics and Computer Science
subject Ashley Benallo, MIT Computer Science and Neuroscience
subjectAudrey Vargas, MIT EECS
subjectDinuri Rupasinghe, MIT AeroAstro
subjectDivya Nori, MIT Computer Science and Biology
subjectDong Young Kim, MIT EECS
subjectHector Martinez, MIT EECS
subjectKristine Zheng, MIT EECS
subjectLara Ozkan, MIT EECS
subjectMichael Hadjiivanov, MIT Mathematics and Computer Science
subjectPreston Hess, MIT EECS
subject Shruti Garg, MIT EECS
subjectSofie Chung, MIT EECS
subjectThelonious Cooper, MIT EECS
Selected undergraduate and high school students with academic positions or scholarships they received
Directly supervised and mentored during and after open source research projects
subject Rijul Magu (CS Ph.D. Program, Georgia Institute of Technology)
subject Gabriela Noemi Gongora (Engineering Management grad program Stevens Institute of Technology)
subject Aditi Mithal (Awarded Google Venkat Panchapakesan Memorial Scholarship, CS grad program at UCLA)
subject Aditi Nath (CS grad program at Arizona State University)
subject Ankita Sastry (Info Security grad program Carnegie Mellon)
subject Karan Rajpal (CS grad program at Cornell University)
subject Nalin Chhibber (Mathematics grad program at the University of Waterloo)
subject Prastut Kumar (The Google Summer of Code Berkman Klein Center Harvard University)
subject Prithvi Raj (Siemens Research and Development, Bangalore)
subject Radhika Bhanu K (CS grad program at Cornell University)
subject Vibhor Sehgal (iSchool Grad program at the University of California, Berkeley)
subject Rahul Sheth (Undergraduate program at the University of California, Los Angeles)
subject William Dai (Undergraduate program at the University of California, Berkeley)
Creativity: Data-rich Installations and Public Exhibitions
Neil's environmental artwork inspires his scientific endeavors in AI and sustainable development. His exhibition Beyond Boundaries captures the complexities of our planet, including 3,000 years old glacier landscapes, the Western Ghats (Sahyadri) of India, cultures, and pressing societal challenges such as climate change. Neil's photography work has been published in National Geographic. For more information, please visit Instagram neilgaikwad_creations CRΞ人TIVΞ PIXΞLS
Beyond Boundaries
Ars Electronica, Festival of Arts, Technology, and Society 2020, As a part of the Virtual Garden Cambridge
MIT Wiesner Art Gallery, 2018
Glacial Place
National Geographic Photo of the Day, 0.1% of submissions
Fostering arts at MIT: Mentoring in arts and tech entrepreneurship
MIT Hacking Arts 2019, MIT’s annual event celebrating the intersection of entrepreneurship, technology, and creative industries
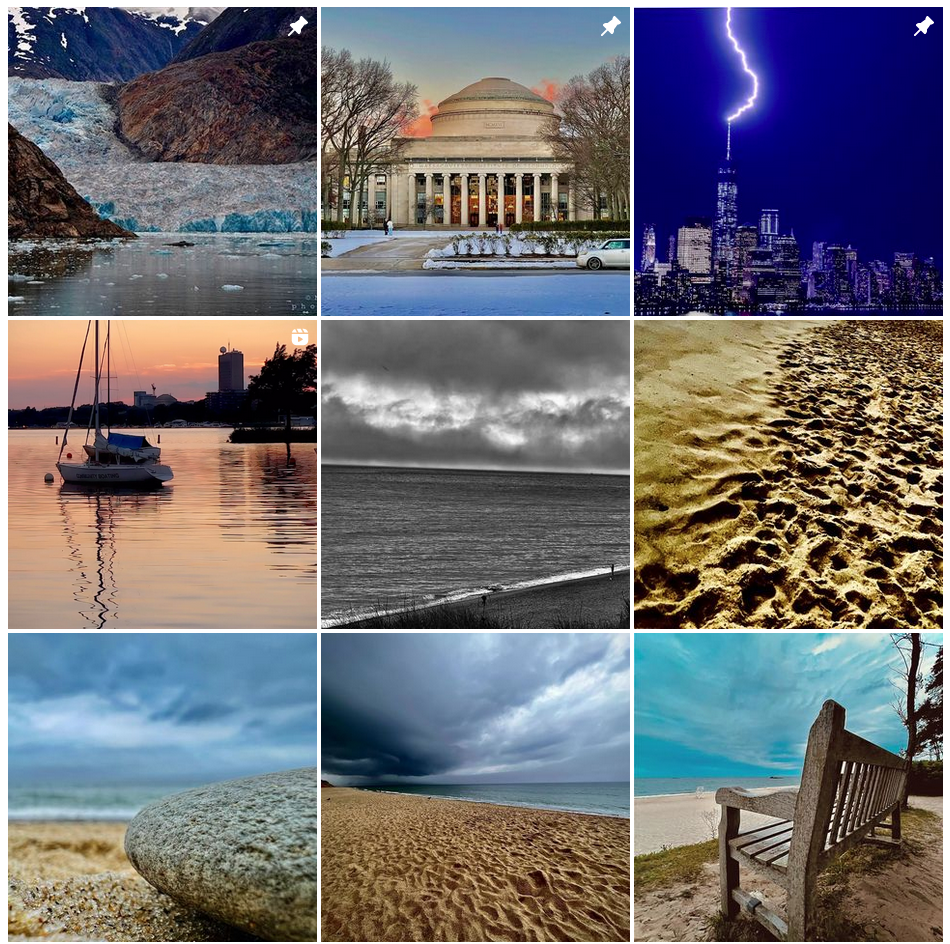
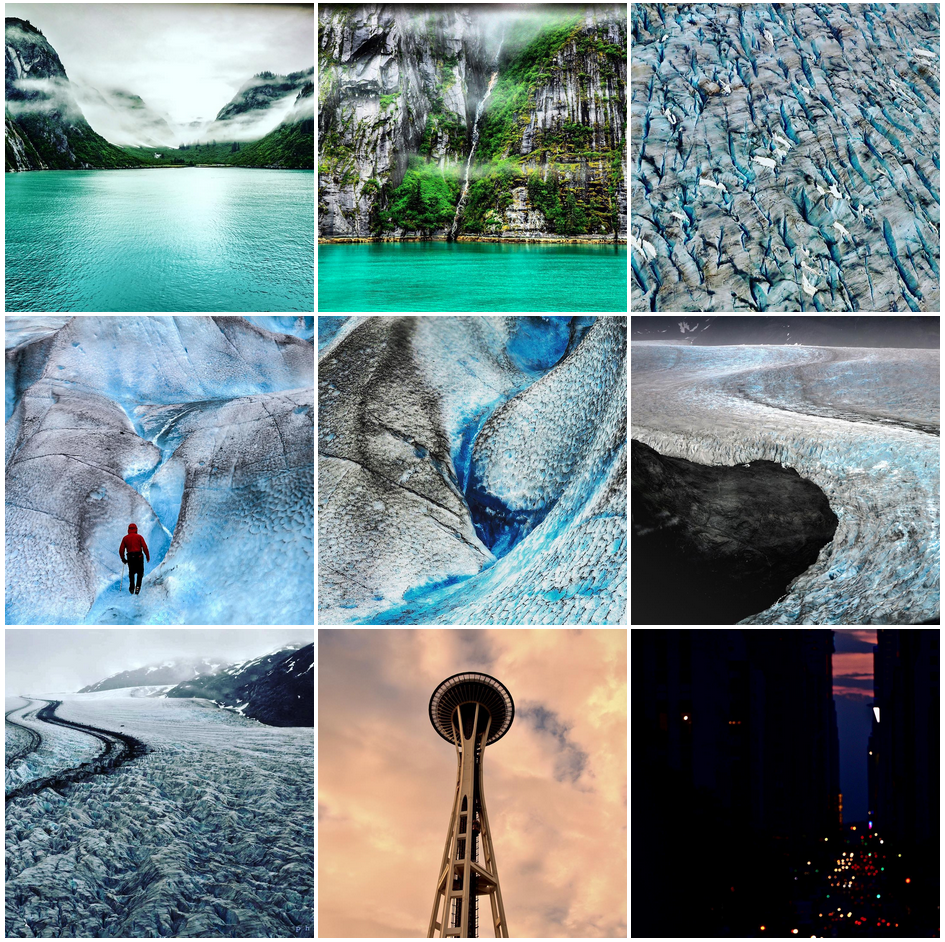
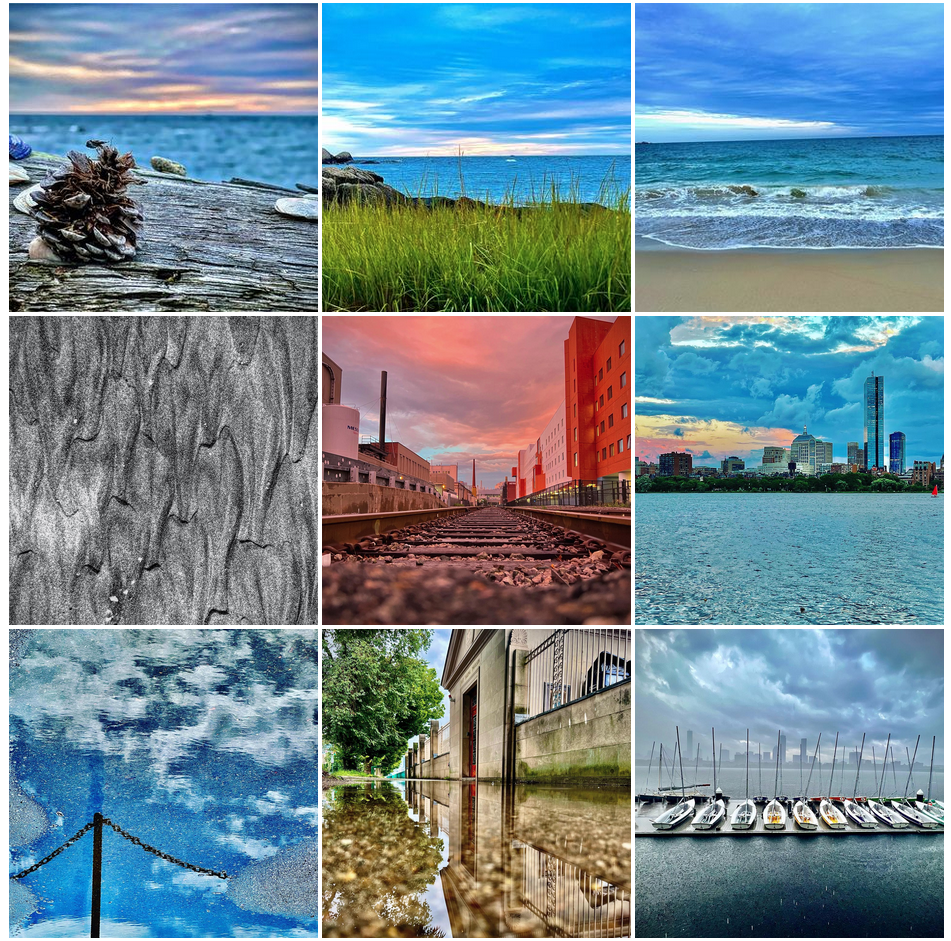
Professional Sport and Research on Team Dynamics
Former professional cricket player---opening batter and leg spinner. Kept wickets (similar to being a catcher in Baseball) for Jermaine Lawson, a former West Indies international cricketer (fast-bowler, 95 miles an hour) and Harshal Patel, India international cricketer fast-bowler.
Some of the archived player of the match scorecards: sports_cricket [146 not out] sports_cricket [71 not out]
Science of Teams (contributions as a research assistant)
Evidence for a Collective Intelligence Factor in the Performance of Human Groups
Science 2010
Anita Woolley, Christopher F. Chabris, Alex Pentland, Nada Hashmi, and Thomas W. Malone
The Effects of Team Strategic Orientation on Team Process and Information Search
Organizational Behavior and Human Decision Processes, 2013
Anita Woolley, Julia B. Bear, Jin Wook Chang, and Arwen Hunter DeCostanza
Where The Mind is Without Fear
Where knowledge is free
Where the world has not been broken up into fragments
By narrow domestic walls
Where words come out from the depth of truth
Where tireless striving stretches its arms towards perfection
Where the clear stream of reason has not lost its way
Into the dreary desert sand of dead habit
Where the mind is led forward by thee
Into ever-widening thought and action
Into that heaven of freedom, my Father, let my country awake.
Nobel Laureate Rabindranath Tagore
The Impossible Dream (The Quest)
To fight the unbeatable foe
To bear with unbearable sorrow
To run where the brave dare not go
To right the unrightable wrong | To love pure and chaste from afar
To try when your arms are too weary | To reach the unreachable star
This is my quest | To follow that star
No matter how hopeless | No matter how far
To fight for the right | Without question or pause
To be willing to march into Hell | For a heavenly cause
And I know if I'll only be true | To this glorious quest
That my heart will lie peaceful and calm | When I'm laid to my rest
And the world will be better for this
That one man, scorned and covered with scars
Still strove with his last ounce of courage
To reach the unreachable star!
Joe Darion (From The Man of La Mancha)